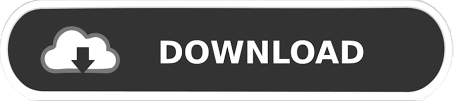
However, it may produce inadmissible solutions on smaller sample sizes and when common factor assumptions do not hold (e.g., the construct follows a composite model e.g., Rigdon 2016 Rigdon, Sarstedt, and Ringle, 2017 Sarstedt et al. It is well-known that this reliability statistic is consistent in PLS-SEM (i.e., it approaches the true reliability under common regularity conditions of a common factor model and when sample sizes increase to infinity). Therefore, it uses a new reliability measure specific to the PLS-SEM context termed rho_A (Dijkstra and Henseler, 2015). The consistent PLS-SEM (PLSc-SEM) algorithm performs a correction of reflective constructs' correlations to make results consistent with a factor-model (Dijkstra and Henseler, 2015 Dijkstra and Schermelleh-Engel, 2014). 2) What are potential problems? When you use the consistent PLS-SEM (PLSc-SEM) bootstrapping: This can lead to incorrect inferences from the model and the data (e.g., faults in the significance assessment). Consequently, the results of the bootstrapping routine may underestimate the actual variability of the parameter estimate (e.g. The result would only consider the "good" bootstrap solutions. In addition, filtering out inadmissible solutions would distort the estimation of the sample distribution generated by bootstrapping. With this information, the user can address the problem in order to create an adequate solution.

The lack of results makes the researchers aware of the problem. Inadmissible solutions always indicate a problem with the data or the model. 1) Why are invalid bootstrapping solutions not filtered out? Note: To replicate the problem shown above, run SmartPLS 3, open the ECSI model, select the data set with 98 observations, and start the consistent bootstrapping with the default settings.
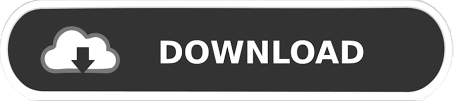